Abstract
In absence of a closed form expression such as in the Heston model, the option pricing is computationally intensive when calibrating a model to market quotes. This article proposes an alternative to standard pricing methods based on physics-inspired neural networks (PINNs). A PINN integrates principles from physics into its learning process to enhance its effciency in solving complex problems.
In this article, the driving principle is the Feynman-Kac (FK) equation, which is a partial differential equation (PDE) governing the derivative price in the Heston model. We focus on the valuation of European options and show that PINNs constitute an efficient alternative for pricing options with various specifications and parameters without the need for retraining.
Keywords: neural networks, variable annuities, Feynman-Kac equation, life insurance.
Sector: Insurance
Expertise: Life Insurance
Authors: Donatien Hainaut, Alex Casas
Publisher: Detralytics
Date: January 2024
Language: English
Pages: 21
Reference : Detra Note 2024-1
About the authors
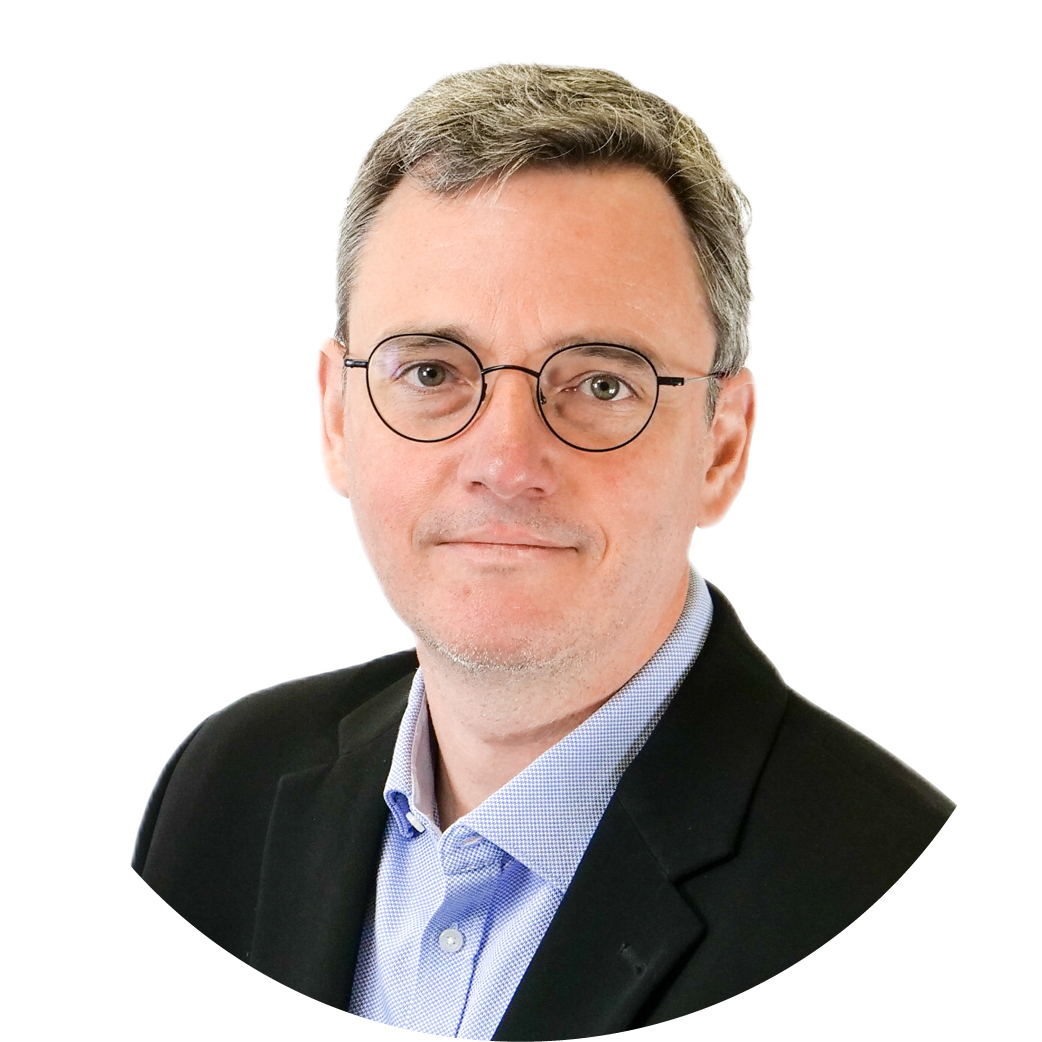
Donatien Hainaut
Donatien Hainaut is a Scientific Advisor at Detralytics and a professor at UCLouvain (Belgium), where he serves as the Director of the Master’s program in Data Science with a statistical orientation. Prior to this, he held several academic positions, including Associate Professor at Rennes School of Business and ENSAE in Paris. He also has extensive industry experience, having worked as a Risk Officer, Quantitative Analyst, and ALM Officer.
Donatien is a Qualified Actuary and holds a PhD in the field of Asset and Liability Management. His current research focuses on contagion mechanisms in stochastic processes and the applications of neural networks in insurance.
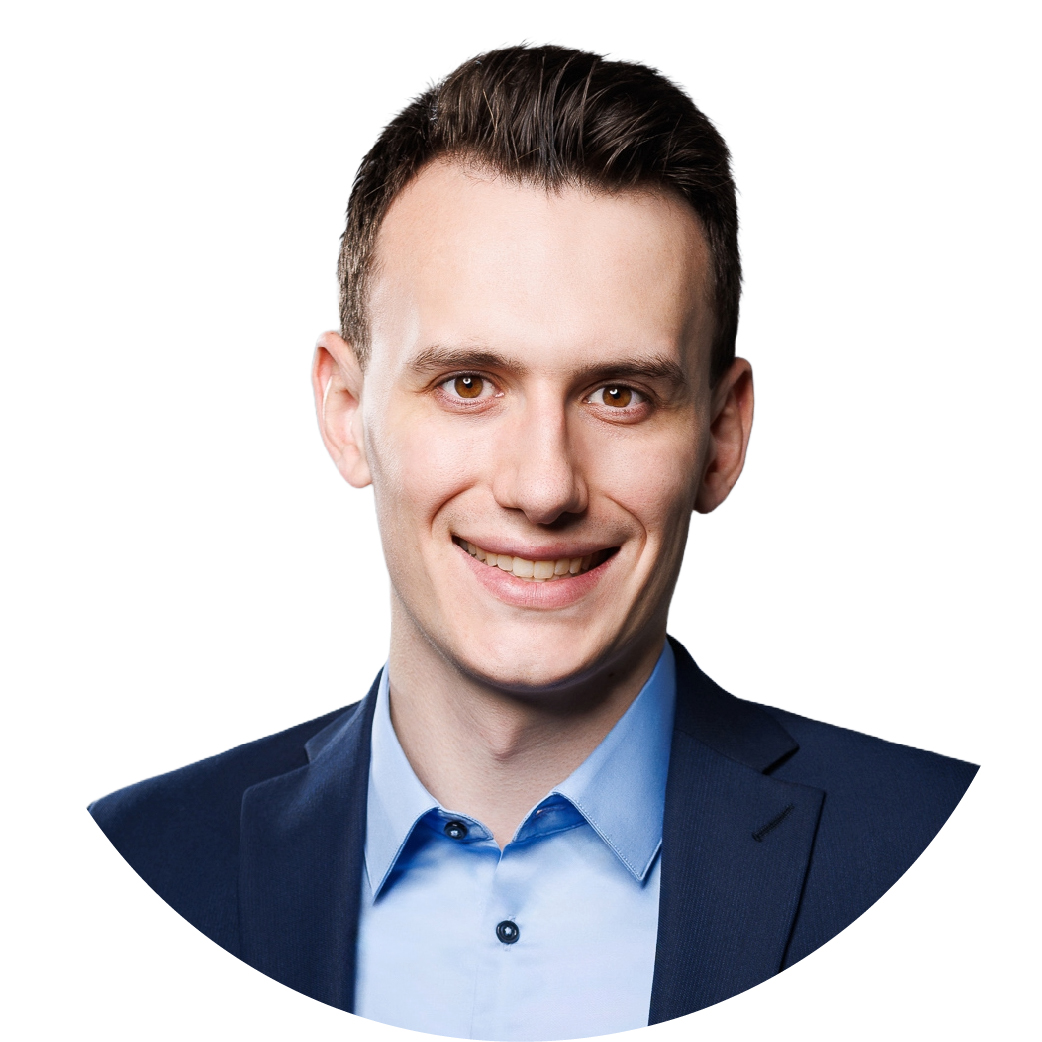
Alex Casas
Alex is part of the Talent Accelerator Program (TAP) at Detralytics. He developed skills in Life and ALM-related subjects during his first experience with a leading bancassurer. Highly proactive and reliable, he will be able to support you in the various missions related to this field.