Abstract
This paper proposes a new approach to risk classification based on Generalized Gaussian Process Regression (GGPR). The response under consideration obeys a distribution belonging to the Exponential Dispersion (ED) family. It typically corresponds to a claim count or a claim severity in the context of insurance studies. GGPR is a supervised machine learning method with Bayesian flavor. Individual random effects obeying a multivariate Normal distribution are connected with the help of their covariance matrix built from a so-called kernel function. The latter enforces smoothness, borrowing information from similar risk profiles. Bayesian Generalized Linear Models (GLMs) and Generalized Additive Models (GAMs) are recovered as special cases, assuming a highly-structured prior covariance matrix. Compared to the existing literature, this paper innovates to account for the specificity of data entering insurance studies. First, proper risk exposures are included in model formulation and development. Second, parameters are estimated by minimizing deviance instead of an approximated log-likelihood. Third, categorical features that are often encountered in insurance data bases are coded with the help of an embedding method based on Burt matrices. Fourth, K-means clustering is used to reduce the dimension of the problem and create model points within large insurance portfolios. Numerical illustrations performed on publicly available insurance data sets illustrate the relevance of the GGPR approach to risk classification. Benchmarked against the classical GLM, the performances of GGPR turn out to be excellent given its reduced number of parameters. This suggests that GGPR nicely enriches the actuarial toolkit by providing preliminary predictions that can then be structured with additive scores like those entering GLMs and GAMs.
Keywords: Exponential Dispersion family, Mixed models, Risk classification, Categorical embedding, Burt distance, Model points.
Sector: Insurance
Expertise: Risk
Authors: Donatien Hainaut and
Michel Denuit
Publisher: Detralytics
Date: April 2025
Language: English
Pages: 34
Reference : Detra Note 2025-2
About the authors
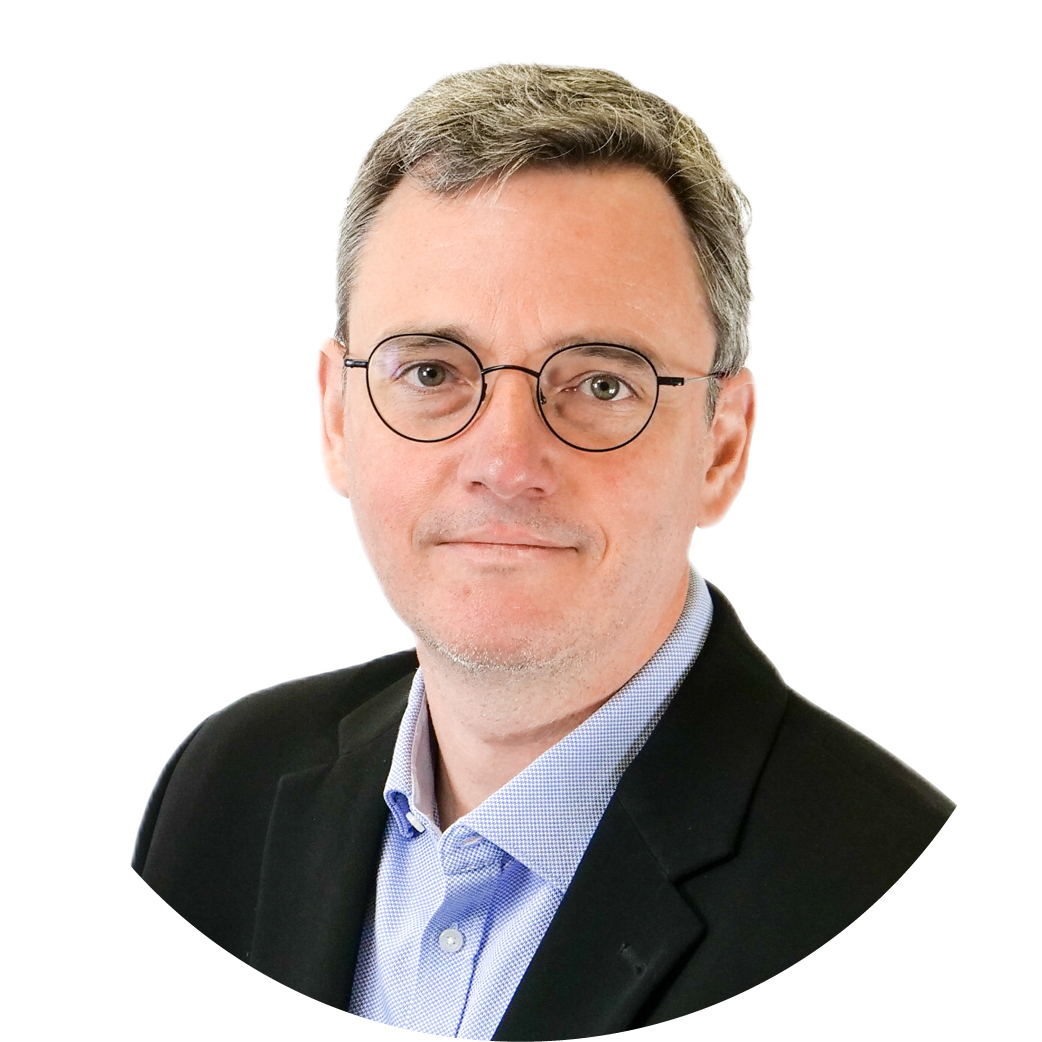
Donatien Hainaut
Donatien Hainaut is a Scientific Advisor at Detralytics and a professor at UCLouvain (Belgium), where he serves as the Director of the Master’s program in Data Science with a statistical orientation. Prior to this, he held several academic positions, including Associate Professor at Rennes School of Business and ENSAE in Paris. He also has extensive industry experience, having worked as a Risk Officer, Quantitative Analyst, and ALM Officer.
Donatien is a Qualified Actuary and holds a PhD in the field of Asset and Liability Management. His current research focuses on contagion mechanisms in stochastic processes and the applications of neural networks in insurance.
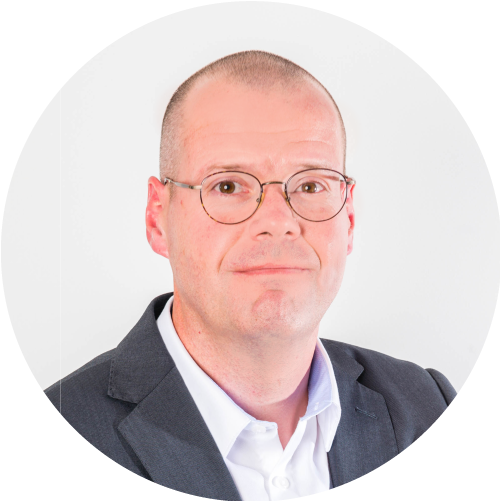
Michel Denuit
Michel is an Honorary Scientific Advisor at Detralytics, as well as a professor in actuarial science at the Université Catholique de Louvain. He has international experience as a visiting professor, and has promoted many projects in collaboration with the industry. At Detralytics, Michel coaches young talents, provides cutting-edge training, fosters innovation and oversees R&D projects.