Abstract
This paper focuses on partial dependence plots which are often used when modeling with machine learning techniques in order to better understand the effects of the features on the conditional expectation of the response variable. However, these plots must be interpreted with caution. Indeed, they can easily lead to wrong interpretations in case the analyst is not enough familiar with these plots. As noticed in a previous FAQctuary, a typical situation is the case where a feature is important because of its interactions with others while its partial dependence plot is flat. In this FAQctuary, we go one step further and we consider a very simple example with a three-way interaction effect and we show that only looking at partial dependence plots for each feature and for two features may indeed lead the analyst to wrong conclusions.
Sector: Insurance
Expertise: Machine learning
Authors: Elke Gagelmans,
Michel Denuit and Julien Trufin
Publisher: Detralytics
Date: November 2020
Language: English
Pages: 5
Reference : FAQctuary 2020-2
About the authors
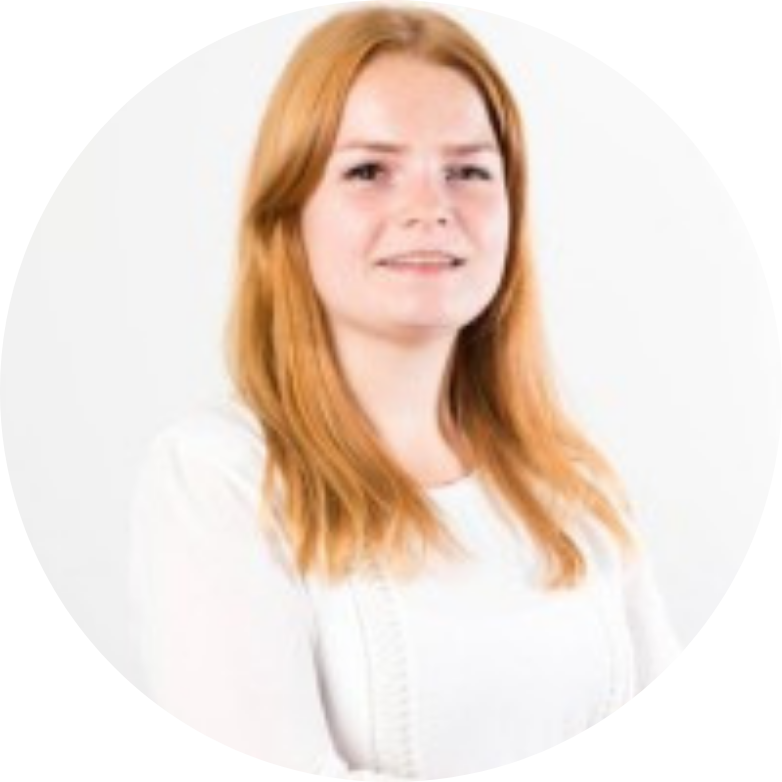
Elke Gagelmans
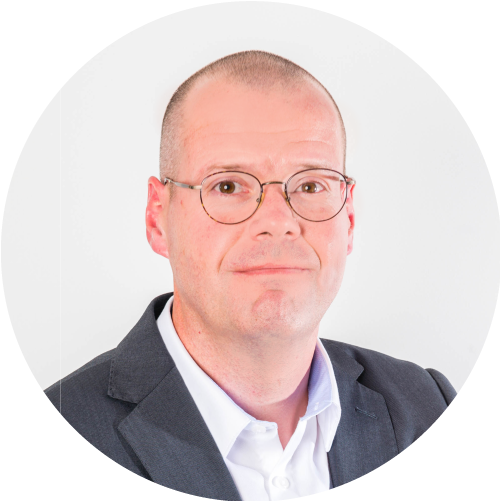
Michel Denuit
Michel is an Honorary Scientific Advisor at Detralytics, as well as a professor in actuarial science at the Université Catholique de Louvain. He has international experience as a visiting professor, and has promoted many projects in collaboration with the industry. At Detralytics, Michel coaches young talents, provides cutting-edge training, fosters innovation and oversees R&D projects.
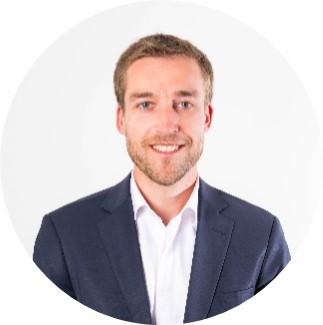
Julien Trufin
Julien is a Scientific Advisor at Detralytics, as well as a professor in Actuarial Science at the department of mathematics of the Université Libre de Bruxelles. Julien has experience as a consultant and a strong academic background developed at prominent institutions, including Université Laval (Canada), UCL, and ULB (Belgium). At Detralytics, Julien coaches young talents, provide cutting-edge training, fosters innovation and oversees R&D projects.