Abstract
Autocalibration is a desirable property, intimately related to the method of marginal totals that predates modern risk classification methods. Autocalibrated mean predictors π for a response Y and feature variables X are such that π(X) =E[Y |π(X)]. Autocalibration is beneficial since it ensures that the information contained in π is used without any systematic errors. Or stated differently, an autocalibrated predictor is optimal with respect to the information contained in it. Autocalibration can easily be implemented using the practical method proposed by Denuit, Charpentier and Trufin (2021), consisting in an extra local regression step. Professional practice favors Lorenz curves and Gini coefficients to assess the performances of a candidate premium. Under autocalibration, Denuit and Trufin (2021) established that the advanced diagnostic tools proposed by Denuit, Sznajder and Trufin (2019) reduce to Lorenz curve and Gini coefficients, respectively. The present note aims to assess the impact of autocalibration on the goodness of lift. Lift graphs compare average predicted and average actual loss costs when policies have been ranked according to the candidate premium and grouped within buckets. It is shown on a case study that autocalibration does not only restore global and local balances but also improve lift.
Keywords: Risk classification, Ratemaking, Lift curve, Goodness of lift, Machine learning.
Sector: Insurance
Expertise: Autocalibration
Authors: Nicolas Ciatto,
Harrison Verelst,
Michel Denuit, and Julien Trufin
Publisher: Detralytics
Date: July 2022
Language: English
Pages: 17
Reference : FAQctuary 2022-2
About the authors
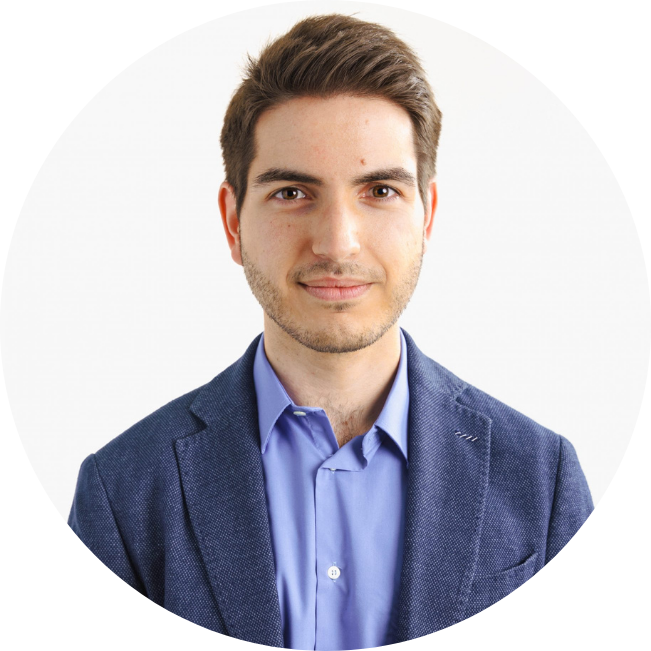
Nicolas Ciatto
Nicolas is part of the Talent Consolidation Program (TCP). He is also People Lead at Detralytics. Prior to joining the company, Nicolas did an actuarial internship at Axa Belgium, in the P&C department. With a strong expertise in non-life insurance and pricing, he worked on the implementation of Non-Life methods in the sight of a priori and a posteriori pricing using R.
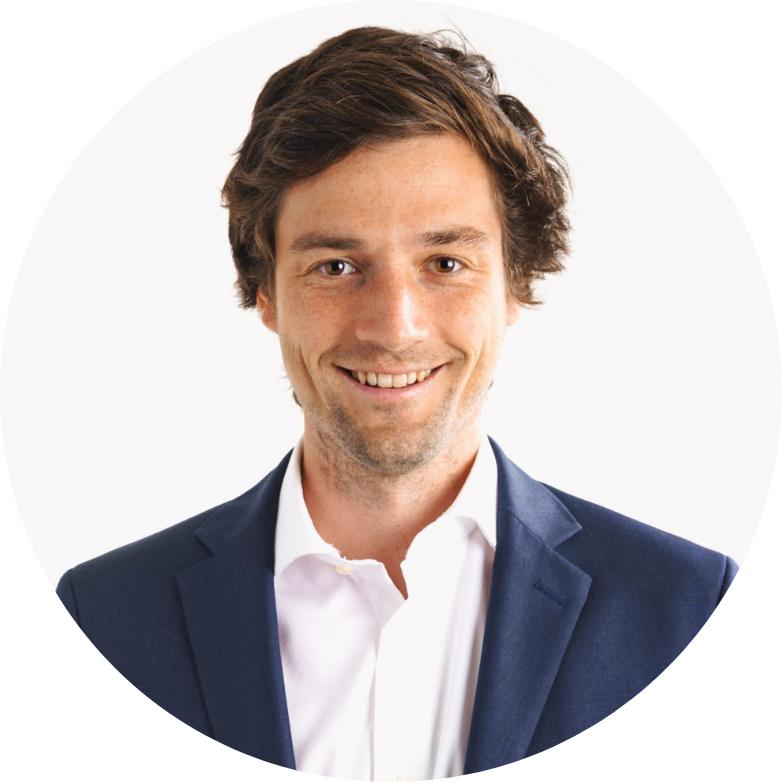
Harrison Verelst
Harrison was part of the Talent Consolidation Program (TCP) at the time of the publication. He holds two Master’s degrees in Mechanical Engineering and Quantitative Finance as well as a Master’s in actuarial sciences from ULB.
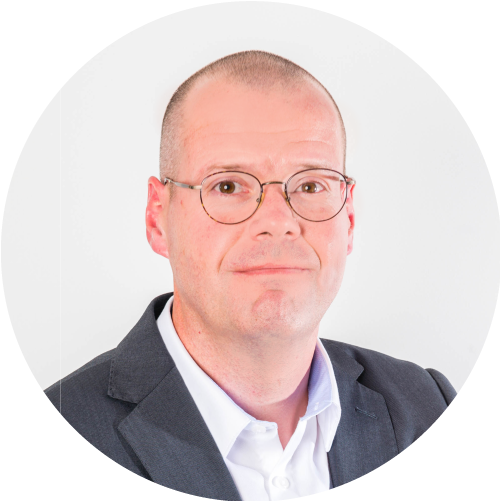
Michel Denuit
Michel is an Honorary Scientific Advisor at Detralytics, as well as a professor in actuarial science at the Université Catholique de Louvain. He has international experience as a visiting professor, and has promoted many projects in collaboration with the industry. At Detralytics, Michel coaches young talents, provides cutting-edge training, fosters innovation and oversees R&D projects.
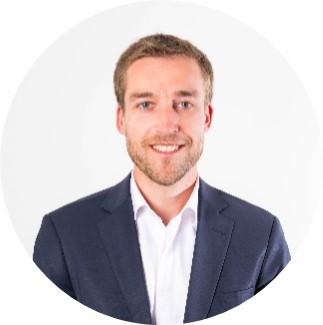
Julien Trufin
Julien is a Scientific Advisor at Detralytics, as well as a professor in Actuarial Science at the department of mathematics of the Université Libre de Bruxelles. Julien has experience as a consultant and a strong academic background developed at prominent institutions, including Université Laval (Canada), UCL, and ULB (Belgium). At Detralytics, Julien coaches young talents, provide cutting-edge training, fosters innovation and oversees R&D projects.